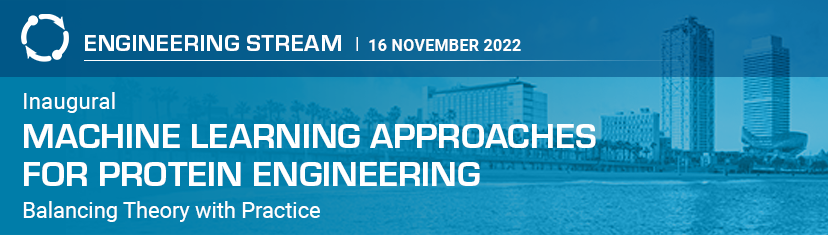
In silico prediction, engineering, and design are changing the way drugs will be discovered, designed, and optimised in the future. These tools are still in their early development and much needs to be learned on how to adapt them for use in antibody and vaccine discovery, training, prediction, developability, simulation, and optimisation.