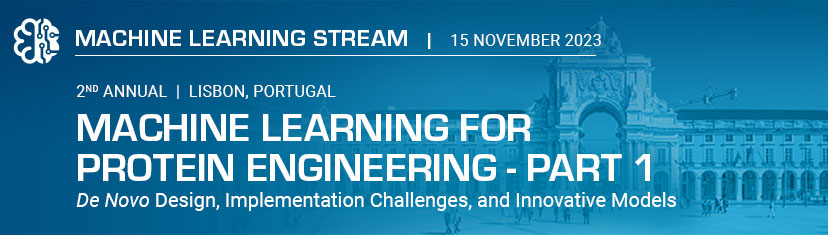
The two-part Machine Learning for Protein Engineering conference at PEGS Europe explores the informatics technologies and strategies driving the improvement of quality, precision and developability of biotherapeutics via the application of artificial intelligence and machine learning. The 2023 meeting will focus on discovery and optimization campaigns via a critical examination of use cases, experimental validation procedures, integrations with legacy discovery platforms and data creation/curation. These programs will offer the PEGS Europe community a forum in which to debate successes to date in adopting this nascent family of technologies – and how research organizations can realize the potential in their significant investments in this space. This conference will demonstrate the considerable value of these techniques and emphasize the practical applications over the purely theoretical exercises. The format will be educational and interactive.